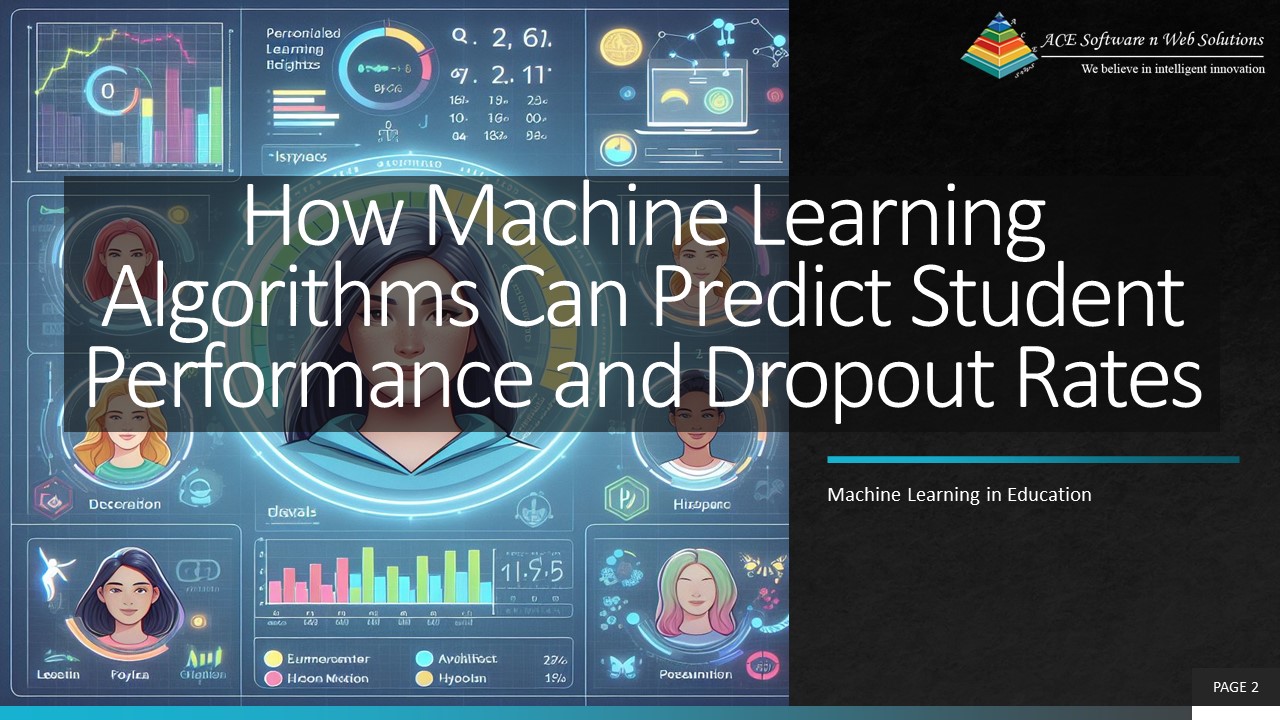
How ML Algorithms Can Predict Student Performance and Dropout Rates
In the educational landscape, the ability to predict student performance and potential dropout risks is invaluable for creating interventions that can lead to more successful outcomes. Machine Learning (ML) algorithms offer powerful tools for educators and decision-makers in the primary and secondary education sectors to understand and act upon these challenges. This blog aims to elucidate how ML algorithms can be employed to predict student performance and dropout rates, offering actionable insights to enhance educational strategies and support student success.
Understanding ML in Educational Contexts
Machine Learning, a subset of Artificial Intelligence (AI), involves the use of algorithms that can learn from and make predictions or decisions based on data. In educational settings, ML can process vast amounts of data regarding student behaviors, academic performance, and other relevant variables to predict future outcomes. Key applications include:
- Predictive Analytics: Using historical and real-time data to forecast student performance and identify those at risk of underperforming or dropping out.
- Personalized Learning: Tailoring educational content and interventions based on individual student needs and learning patterns.
Predicting Student Performance
Early Identification of At-Risk Students
By analyzing data such as grades, attendance records, engagement levels, and more, ML algorithms can identify students who are at risk of falling behind. This allows educators to intervene early with targeted support, such as:
- Tutoring and mentoring programs.
- Customized learning materials.
- Enhanced engagement strategies.
Enhancing Academic Outcomes
ML can help tailor the learning experience for each student, leading to improved academic outcomes. This involves:
- Adapting curriculum pacing according to the student’s learning speed.
- Providing personalized feedback and recommendations.
- Predicting and addressing areas where students may face challenges.
Predicting Dropout Rates
Identifying Predictive Factors
ML algorithms can analyze a wide range of factors that may contribute to a student’s likelihood of dropping out, such as:
- Socioeconomic status.
- Academic performance and progression.
- Engagement with school activities.
- Attendance and behavioral patterns.
By understanding these factors, schools can develop more effective strategies to retain students.
Targeted Interventions
With insights from ML predictions, schools can implement targeted interventions to reduce dropout rates, including:
- Academic support and counseling services.
- Engagement and extracurricular programs.
- Family and community involvement initiatives.
Implementing ML in Schools
For ML algorithms to effectively predict student performance and dropout rates, several key steps must be taken:
- Data Collection and Management: Establishing robust systems for collecting and managing diverse data sets related to student performance and engagement.
- Collaboration with ML Experts: Working with ML professionals to develop and deploy predictive models tailored to the school’s specific needs.
- Ethical Considerations and Transparency: Ensuring the ethical use of student data and maintaining transparency about how ML predictions are used to support student outcomes.
- Continuous Improvement: Regularly reviewing and refining ML models and interventions based on outcomes and feedback.
The Future of ML in Education
As ML technology continues to evolve, its potential to transform educational strategies and outcomes grows. Future advancements may enable even more accurate predictions and personalized interventions, ultimately leading to higher student success rates and lower dropout rates. Innovations could include:
- Advanced analytics for real-time interventions.
- Integration with immersive learning technologies for engaging educational experiences.
- Enhanced predictive models that incorporate broader social and emotional factors.
In conclusion, ML algorithms offer a promising avenue for enhancing educational outcomes by predicting student performance and identifying those at risk of dropping out. By leveraging these technologies, educators and decision-makers can implement targeted interventions to support student success. As we look to the future, the continued integration of ML in education will play a crucial role in fostering an environment where every student has the opportunity to thrive.