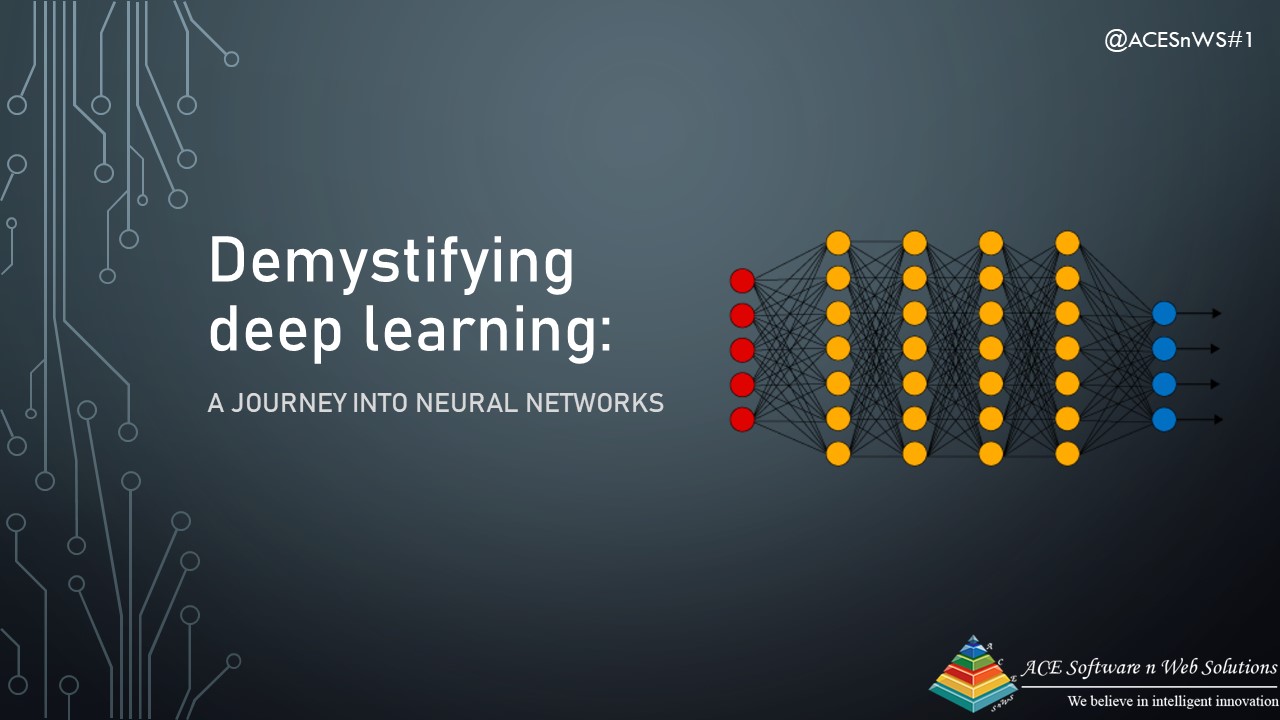
Demystifying Deep Learning: A Journey into Neural Networks
Deep learning and neural networks have become buzzwords in the world of artificial intelligence and machine learning. They power a wide range of applications, from image recognition to natural language processing. But what exactly are neural networks, and how do they work? In this blog post, we’ll take you on a journey to demystify deep learning and explore the inner workings of neural networks.
Understanding the Basics
At its core, deep learning is a subset of machine learning that mimics the way the human brain processes information. Neural networks, the building blocks of deep learning, are inspired by the structure and function of biological neurons. These networks consist of layers of interconnected nodes, or artificial neurons, which work together to process data and make predictions.
Layers in Neural Networks
Neural networks typically consist of three main types of layers:
- Input Layer: This layer receives raw data or features from the outside world. Each neuron in the input layer corresponds to a specific input feature.
- Hidden Layers: Between the input and output layers, there can be one or more hidden layers. These layers are responsible for learning patterns and relationships within the data.
- Output Layer: The final layer provides the network’s predictions or classifications based on the input and what the hidden layers have learned.
How Neural Networks Learn
Neural networks learn through a process called training. During training, the network is exposed to a dataset with known inputs and corresponding outputs. The network’s goal is to adjust its internal parameters, or weights, so that it can accurately predict the outputs for a given set of inputs.
Here’s a simplified overview of the training process:
- Forward Propagation: The input data is fed through the network, and the network’s predictions are calculated. Any differences between the predictions and the actual outputs are measured using a loss function.
- Backpropagation: The network uses the calculated loss to adjust the weights in the reverse direction, from the output layer back to the input layer. This process helps the network learn how to make more accurate predictions.
- Repeating the Process: The forward and backward passes are repeated for many iterations, gradually improving the network’s ability to make accurate predictions.
Deep Learning’s Power
The term “deep learning” comes from the use of deep neural networks, which have multiple hidden layers. Deeper networks can learn complex and abstract features from data, making them suitable for a wide range of tasks, including image and speech recognition.
Real-World Applications
Deep learning and neural networks are behind many of the AI applications we encounter daily:
- Image Recognition: Convolutional Neural Networks (CNNs) excel at recognizing objects and patterns in images.
- Natural Language Processing: Recurrent Neural Networks (RNNs) and Transformer models are used for tasks like language translation, sentiment analysis, and chatbots.
- Autonomous Vehicles: Deep learning is crucial for self-driving cars, helping them detect and navigate their surroundings.
Challenges and Future
While deep learning has achieved remarkable success, it’s not without its challenges. Issues like overfitting, interpretability, and ethical concerns need to be addressed as the field continues to evolve. However, the potential for deep learning to revolutionize industries and improve our lives is immense.
In the coming years, we can expect more breakthroughs in deep learning, with applications expanding into areas we can’t yet imagine. As we continue our journey into the world of neural networks, one thing is clear: deep learning is a powerful tool that will shape the future of AI.
Conclusion
Deep learning and neural networks are at the forefront of AI innovation. By understanding their basic principles and inner workings, we can better appreciate their potential and the incredible applications they power. As we demystify deep learning, we open the door to new possibilities and a brighter future for AI.
So, whether you’re a seasoned data scientist or just starting your AI journey, neural networks are a fascinating subject to explore. Keep learning, experimenting, and stay curious about the limitless possibilities of deep learning.