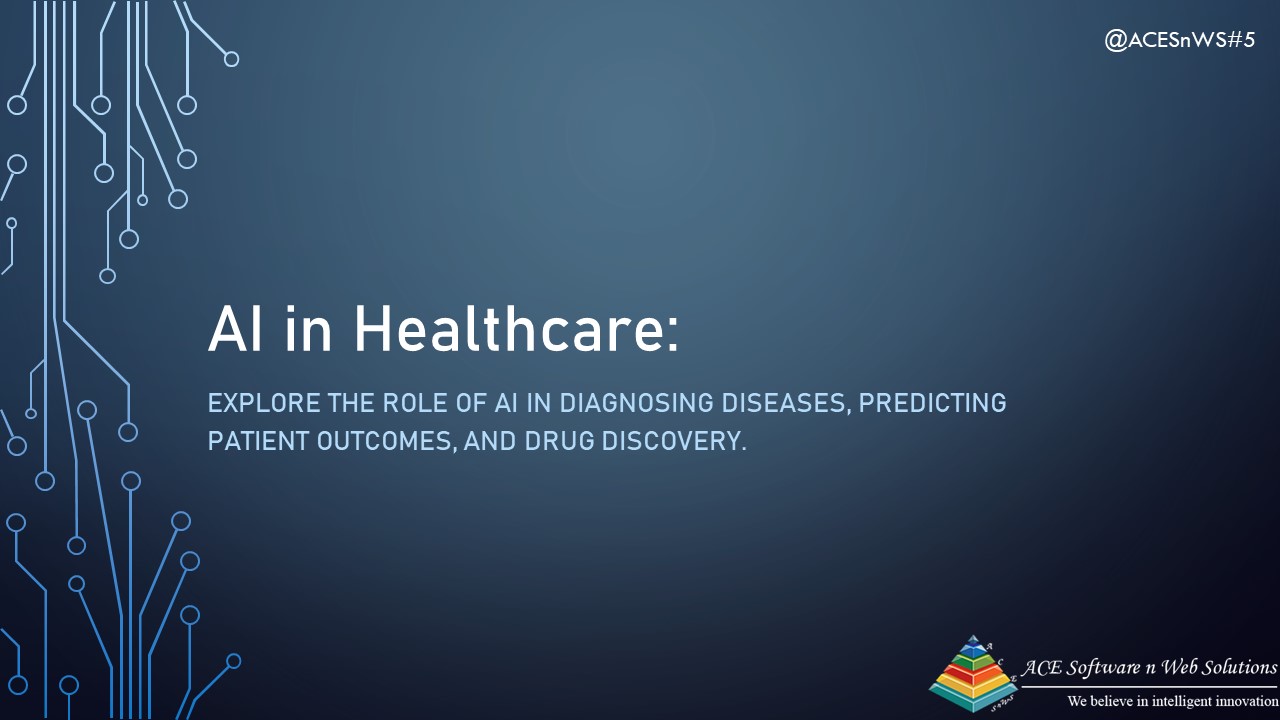
The Healing Power of AI: Revolutionizing Disease Diagnosis, Patient Outcomes Prediction, and Drug Discovery
Introduction
Artificial Intelligence (AI) has emerged as a powerful tool in the field of healthcare. It’s transforming the way we diagnose diseases, predict patient outcomes, and accelerate the process of drug discovery. In this blog, we’ll explore the multifaceted role of AI in these critical healthcare domains, and how it is reshaping the landscape of medical research and treatment.
AI in Diagnosing Diseases
1. Early Disease Detection: AI algorithms, particularly in radiology, assist in detecting diseases at early stages. For example, deep learning models can identify abnormalities in medical images like X-rays and MRIs, aiding in the early diagnosis of conditions such as cancer, fractures, and neurological disorders.
2. Pathology and Histology: AI-driven image analysis in pathology is helping pathologists identify cancerous cells more accurately. Automated image processing speeds up diagnosis, improves accuracy, and reduces human error.
3. Virtual Health Assistants: AI-powered virtual assistants can analyze a patient’s symptoms and medical history, aiding in diagnosing common ailments or providing initial guidance before consulting a physician.
4. Genetic Diagnostics: AI is employed in analyzing genetic data to identify genetic variations and their links to disease susceptibility. This helps in early intervention and personalized treatment.
Advantages:
- Efficiency: AI can analyze vast amounts of data quickly, reducing the time required for diagnosis.
- Accuracy: Machine learning models are capable of achieving high accuracy levels in identifying diseases.
- Early Intervention: Early detection means better treatment outcomes and lower healthcare costs.
Challenges:
- Data Quality: AI models rely on high-quality, diverse datasets, which can be challenging to obtain.
- Interpretable Models: Explaining AI-driven diagnostic decisions is essential for building trust in medical professionals and patients.
AI in Predicting Patient Outcomes
1. Prognostic Models: AI constructs prognostic models that predict a patient’s future health status based on current data. These models are valuable in conditions such as cancer, where predicting disease progression is crucial for treatment planning.
2. Readmission Risk: AI can identify patients at risk of hospital readmission by analyzing historical data, including previous hospitalizations, medications, and vital signs. This information helps healthcare providers allocate resources efficiently.
3. Personalized Treatment Plans: AI aids in creating individualized treatment plans by considering a patient’s medical history, genetics, and lifestyle. This approach improves treatment efficacy and minimizes side effects.
4. Remote Monitoring: Wearable devices and AI-driven monitoring systems track patient vital signs and symptoms in real-time, allowing for early intervention and reducing hospital visits.
Advantages:
- Personalized Care: Predictive models enable tailored treatment plans, improving patient outcomes.
- Resource Allocation: Hospitals can allocate resources more efficiently by focusing on patients at high risk.
- Reduced Costs: Preventing readmissions and providing early intervention reduces healthcare costs.
Challenges:
- Data Privacy: Patient data security and privacy must be a priority in the use of AI for patient outcome prediction.
- Ethical Concerns: Ensuring that AI-driven predictions do not perpetuate bias or discrimination is essential.
AI in Drug Discovery
1. Drug Screening: AI accelerates drug discovery by screening vast chemical libraries to identify potential drug candidates. It predicts how molecules will interact with target proteins, saving time and resources.
2. Target Identification: AI helps identify potential drug targets by analyzing biological data, including genomics, proteomics, and structural biology.
3. Drug Repurposing: AI can discover new therapeutic uses for existing drugs by analyzing their interactions with different biological pathways. This approach can rapidly bring drugs to market.
4. Clinical Trial Optimization: Predictive modeling can optimize clinical trial designs, reducing the time and cost of bringing new drugs to market.
Advantages:
- Speed: AI-driven drug discovery significantly shortens the time required for the development of new medications.
- Cost Efficiency: Reduced resources are needed for laboratory experiments and clinical trials.
- Personalized Medicine: AI enables the development of drugs tailored to an individual’s genetic makeup.
Challenges:
- Data Availability: Access to comprehensive and high-quality data is essential for the success of AI in drug discovery.
- Validation: AI-driven drug candidates must undergo rigorous validation and clinical trials.
Conclusion
Artificial Intelligence has firmly established its presence in healthcare, transforming the way we diagnose diseases, predict patient outcomes, and discover new drugs. The AI-driven revolution in healthcare offers the promise of earlier diagnoses, more effective treatments, and a brighter future for medical research and patient care. However, addressing challenges such as data privacy, ethical concerns, and the validation of AI-driven predictions remains crucial for the responsible and beneficial use of AI in the healthcare sector.